VSEGAN: Visual Speech Enhancement Generative Adversarial Network
Speech enhancement is an essential task of improving speech quality in noise scenario. Several state-of-the-art approacheshave introduced visual information for speech enhancement, since the visual aspect of speech is essentially unaffected by acoustic environment. This paper proposes a novel frameworkthat involves visual information for speech enhancement, by in-corporating a Generative Adversarial Network (GAN). In par-ticular, the proposed visual speech enhancement GAN consistof two networks trained in adversarial manner, i) a generator that adopts multi-layer feature fusion convolution network to enhance input noisy speech, and ii) a discriminator that attempts to minimize the discrepancy between the distributions of the clean speech signal and enhanced speech signal. Experiment results demonstrated superior performance of the proposed modelagainst several state-of-the-art models.
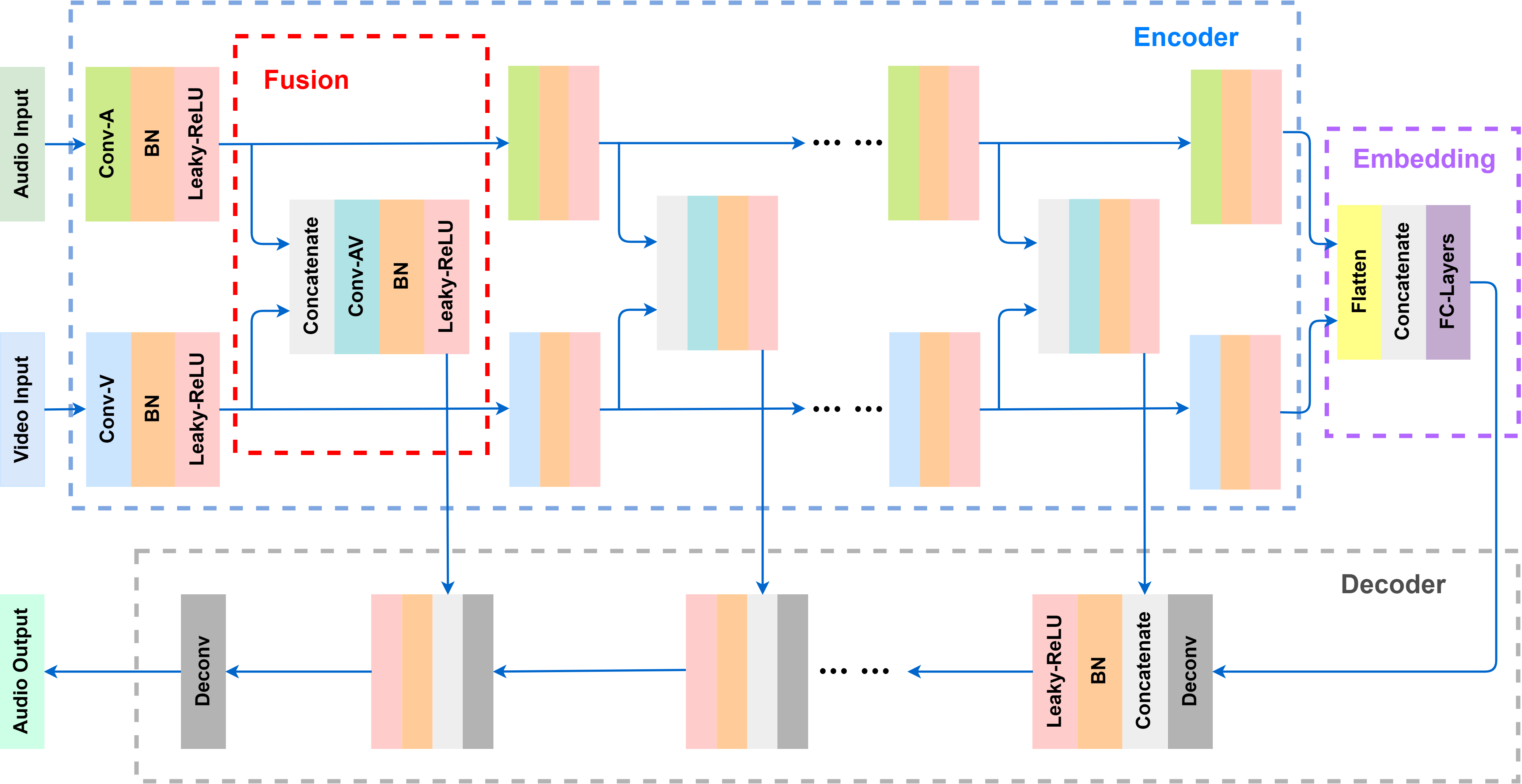
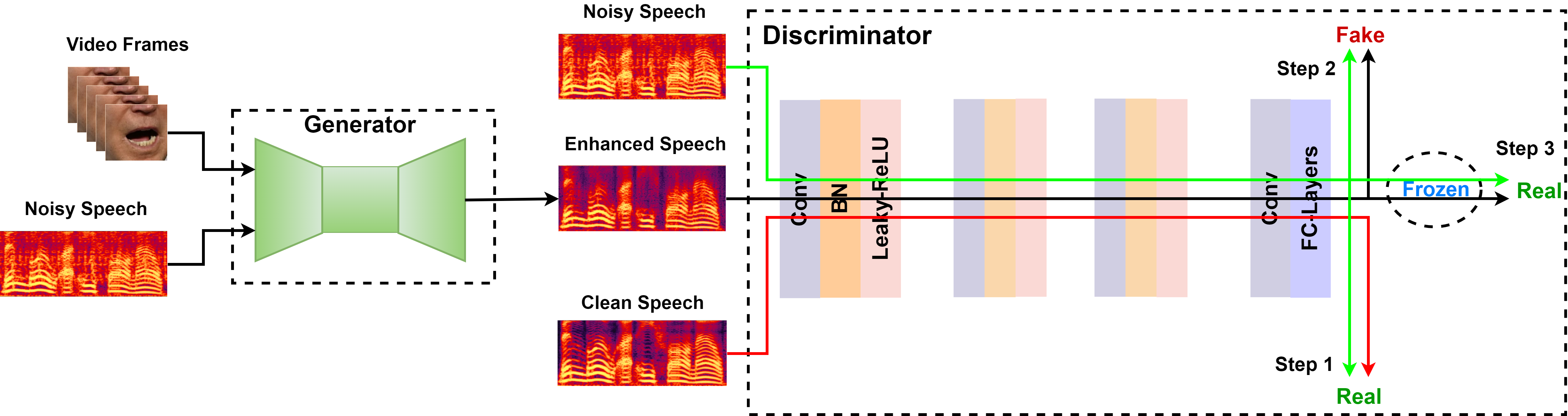
Dataset
- We used the TCD-TIMIT dataset and GRID Corpus dataset.
Experiment Results
Male speech + ambient noise
Noisy | Enhanced-Baseline | Enhanced-VSEGAN-G | Enhanced-VSEGAN | |
---|---|---|---|---|
Sample |
Female speech + ambient noise
Noisy | Enhanced-Baseline | Enhanced-VSEGAN-G | Enhanced-VSEGAN | |
---|---|---|---|---|
Sample |
Female speech + unknown talker speech
Mixture | Enhanced-Baseline | Enhanced-VSEGAN-G | Enhanced-VSEGAN | |
---|---|---|---|---|
Sample |
Male speech + unknown talker speech
Mixture | Enhanced-Baseline | Enhanced-VSEGAN-G | Enhanced-VSEGAN | |
---|---|---|---|---|
Sample |
NOTE:
- If you want to cite this paper, try this: Xinmeng Xu, Yang Wang, Dongxiang Xu, Cong Zhang, Yiyuan Peng, Jie Jia, Binbin Chen, "VSEGAN: Visual Speech Enhancement Generative Adversarial Network"